Chapter
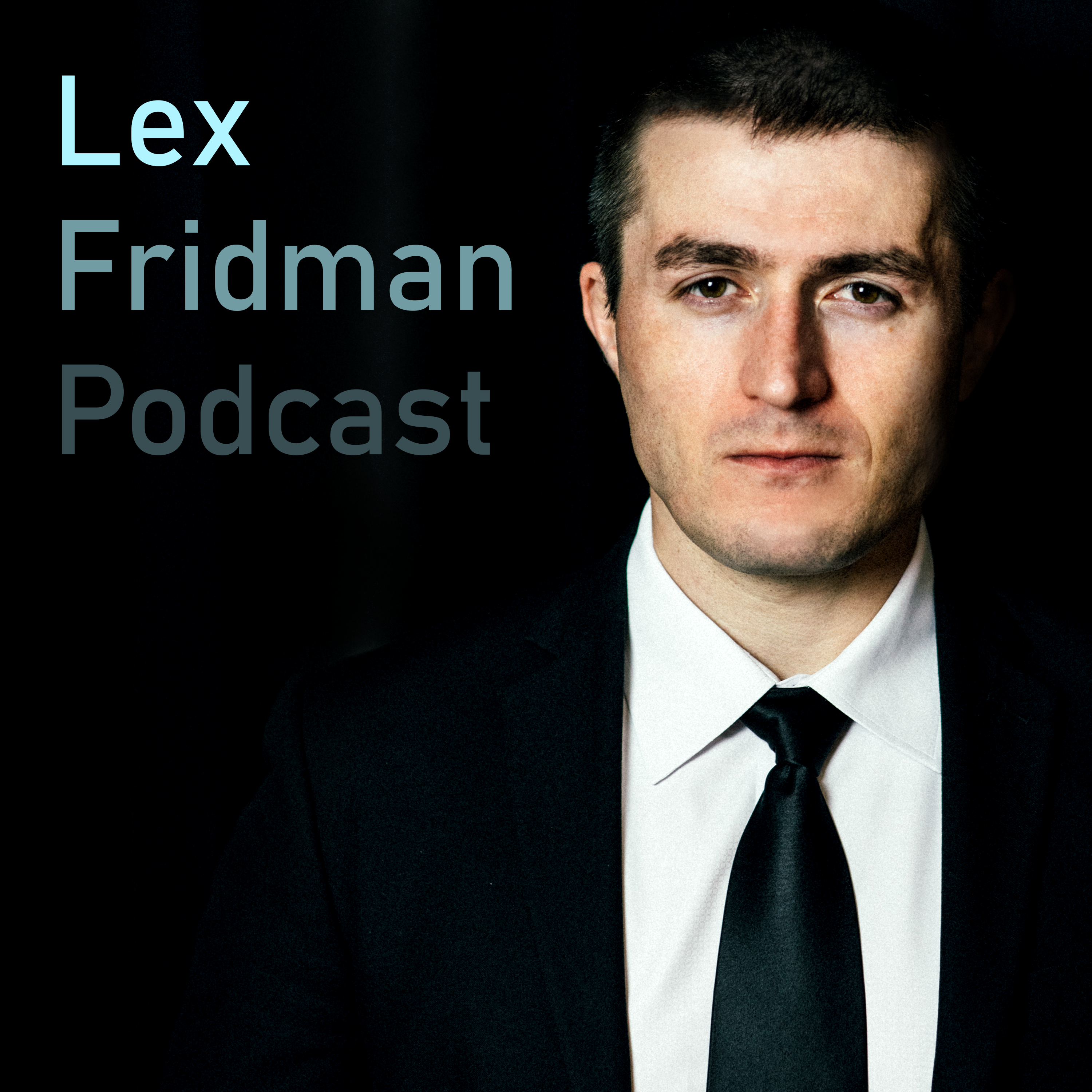
Challenges with Self-Supervised Learning in NLP
The current approaches to self-supervised learning in NLP are oversimplified and do not adequately represent uncertainty, multiple outcomes, or a continuum of possibilities in the distribution of words, leading to a need for a more compressed representation.
Clips
The difficulty with self-supervised learning lies in representing uncertainty, multiple outcomes and unpredictability.
16:59 - 19:37 (02:38)
Summary
The difficulty with self-supervised learning lies in representing uncertainty, multiple outcomes and unpredictability. Although vision appears to be less challenging than language for achieving full self-supervised learning capability, the challenges still remain vast.
ChapterChallenges with Self-Supervised Learning in NLP
Episode#258 – Yann LeCun: Dark Matter of Intelligence and Self-Supervised Learning
PodcastLex Fridman Podcast
The future of natural language processing lies in finding a more compressed representation of word distribution and training algorithms to understand the infinite number of plausible continuations of multiple frames in a high dimensional continuous space.
19:37 - 21:19 (01:41)
Summary
The future of natural language processing lies in finding a more compressed representation of word distribution and training algorithms to understand the infinite number of plausible continuations of multiple frames in a high dimensional continuous space.
ChapterChallenges with Self-Supervised Learning in NLP
Episode#258 – Yann LeCun: Dark Matter of Intelligence and Self-Supervised Learning
PodcastLex Fridman Podcast
Current approaches to self-supervisioning in NLP are limited by the Independent distributions of words and a lack of proper representation of distributions over combinatorial sequences of symbols, which grow exponentially with the length of the symbols.
21:19 - 23:27 (02:08)
Summary
Current approaches to self-supervisioning in NLP are limited by the Independent distributions of words and a lack of proper representation of distributions over combinatorial sequences of symbols, which grow exponentially with the length of the symbols.