Chapter
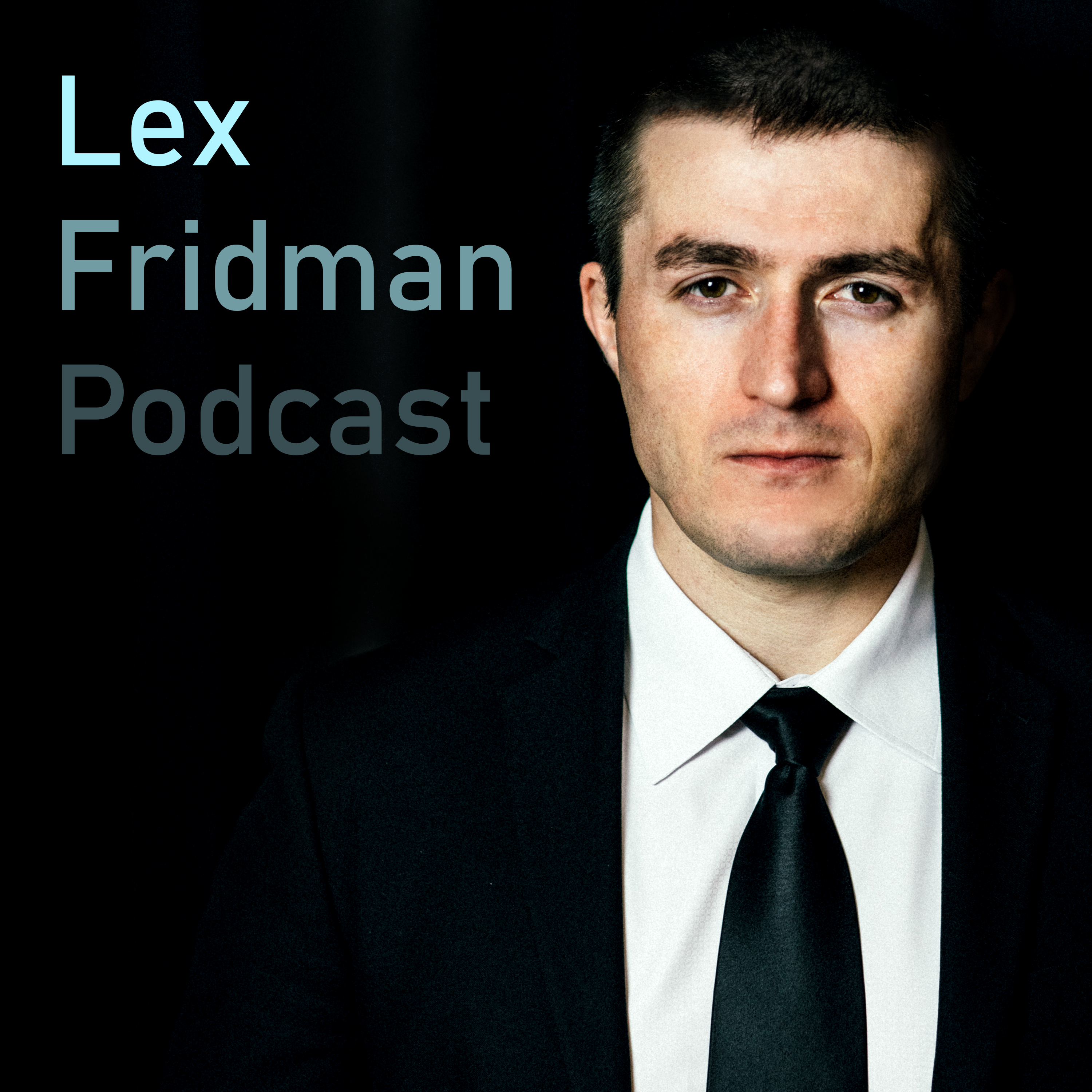
General Problem Solving Machines and Artificial Curiosity
The idea of creating general problem-solving machines involves developing artificial curiosity systems that go beyond current knowledge to become more general problem solvers and understand more of the world. As part of this process, curiosity is a natural feature of the recurrent network that learns to solve problems in a more efficient manner by creating little subnetworks that stand for the properties of the agent, hand, and other actuators.
Clips
The concept of being curious and using experimentation for survival applies not only to official scientists but also babies, who use their toys to understand how the world works.
36:03 - 38:26 (02:22)
Summary
The concept of being curious and using experimentation for survival applies not only to official scientists but also babies, who use their toys to understand how the world works. Curiosity is an important trait humans have, as it allows us to explore and create situations that transcend our existing knowledge base and become more successful problem-solvers.
ChapterGeneral Problem Solving Machines and Artificial Curiosity
EpisodeJuergen Schmidhuber: Godel Machines, Meta-Learning, and LSTMs
PodcastLex Fridman Podcast
The podcast discusses the significance of curiosity and creativity when it comes to solving problems with the help of a problem-solving machine.
38:26 - 41:30 (03:03)
Summary
The podcast discusses the significance of curiosity and creativity when it comes to solving problems with the help of a problem-solving machine. It highlights the difference between applied creativity and pure creativity, the latter being essential to human-level intelligence.
ChapterGeneral Problem Solving Machines and Artificial Curiosity
EpisodeJuergen Schmidhuber: Godel Machines, Meta-Learning, and LSTMs
PodcastLex Fridman Podcast
Recurrent networks are likely to form subnetworks that encode the properties of the agents, hands, and other sensors, to compress and better decode data influenced by the actions of agents, maximising the reward in a given environment, finding the charging station in time while avoiding painful obstacles, and using predictive modelling to plan ahead.
41:30 - 45:57 (04:26)
Summary
Recurrent networks are likely to form subnetworks that encode the properties of the agents, hands, and other sensors, to compress and better decode data influenced by the actions of agents, maximising the reward in a given environment, finding the charging station in time while avoiding painful obstacles, and using predictive modelling to plan ahead.
ChapterGeneral Problem Solving Machines and Artificial Curiosity
EpisodeJuergen Schmidhuber: Godel Machines, Meta-Learning, and LSTMs
PodcastLex Fridman Podcast
The speaker discusses the use of Recurrent Neural Networks (RNNs) and Long Short-Term Memory Networks (LSTMs) in speech recognition, explaining that RNNs are expanded and old versions of a record network, while LSTMs are a type of RNN that allows for better memory retention.
45:57 - 49:27 (03:30)
Summary
The speaker discusses the use of Recurrent Neural Networks (RNNs) and Long Short-Term Memory Networks (LSTMs) in speech recognition, explaining that RNNs are expanded and old versions of a record network, while LSTMs are a type of RNN that allows for better memory retention.