Chapter
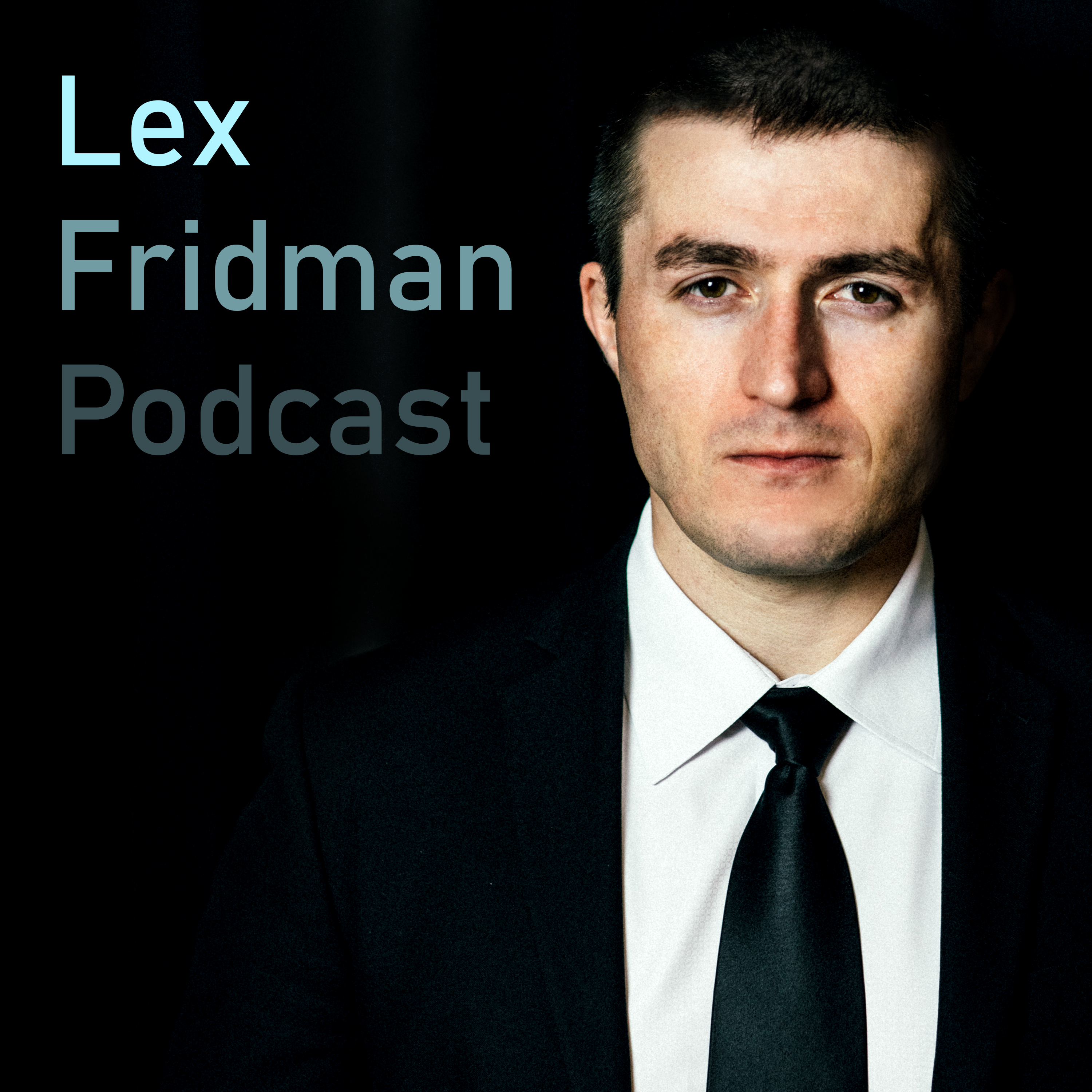
Improving Performance of Machine Learning with Unlabeled Data
Machine learning systems can perform with higher accuracy if trained with millions of unlabeled data, achieving the same level of performance as supervised systems with fewer samples; this advancement could benefit medical image analysis.
Clips
Semi-supervised learning can significantly decrease the number of samples needed to reach a certain level of performance, by using a large amount of unlabeled data in conjunction with a smaller amount of labeled data to train a model.
56:48 - 59:40 (02:52)
Summary
Semi-supervised learning can significantly decrease the number of samples needed to reach a certain level of performance, by using a large amount of unlabeled data in conjunction with a smaller amount of labeled data to train a model.
ChapterImproving Performance of Machine Learning with Unlabeled Data
EpisodeYann LeCun: Deep Learning, Convolutional Neural Networks, and Self-Supervised Learning
PodcastLex Fridman Podcast
The guest speaker expresses skepticism about the effectiveness of active learning in achieving significant breakthroughs in artificial intelligence but acknowledges that it could still be beneficial in certain scenarios with large data sets.
59:41 - 1:00:41 (00:59)
Summary
The guest speaker expresses skepticism about the effectiveness of active learning in achieving significant breakthroughs in artificial intelligence but acknowledges that it could still be beneficial in certain scenarios with large data sets.