Chapter
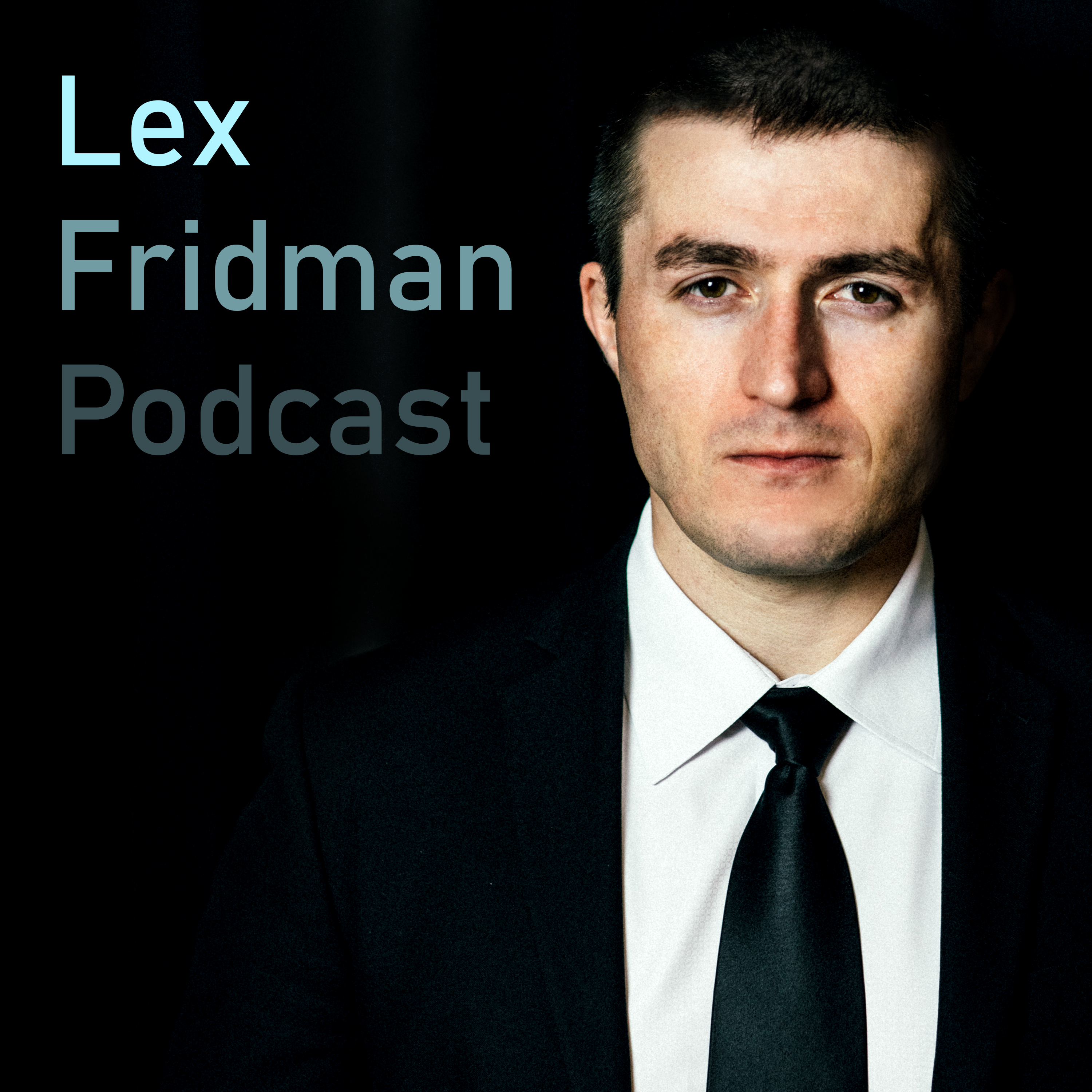
The Benefits of Stochasticity in High Dimensions
The properties of stochasticity are appealing in high dimensions for the law of large number reasons. Stochasticity can save us from some of the particular features of surfaces that are discontinuous in a first derivative, which creates issues with only using gradients.
Clips
Stochasticity in high dimensions has properties that make it appealing for use in algorithms, particularly for surfaces with non-differentiable points.
1:13:58 - 1:16:32 (02:33)
Summary
Stochasticity in high dimensions has properties that make it appealing for use in algorithms, particularly for surfaces with non-differentiable points. Empirically, stochastic gradient has been found to be effective and theory has followed suit.
ChapterThe Benefits of Stochasticity in High Dimensions
Episode#74 – Michael I. Jordan: Machine Learning, Recommender Systems, and the Future of AI
PodcastLex Fridman Podcast
The use of gradient optimization in machine learning dates back 150 years, and while it is important to understand the intuition behind it, it is not enough to fully comprehend how it works.
1:16:32 - 1:19:47 (03:14)
Summary
The use of gradient optimization in machine learning dates back 150 years, and while it is important to understand the intuition behind it, it is not enough to fully comprehend how it works. Using a computer to store previous gradients can optimize this process.