Chapter
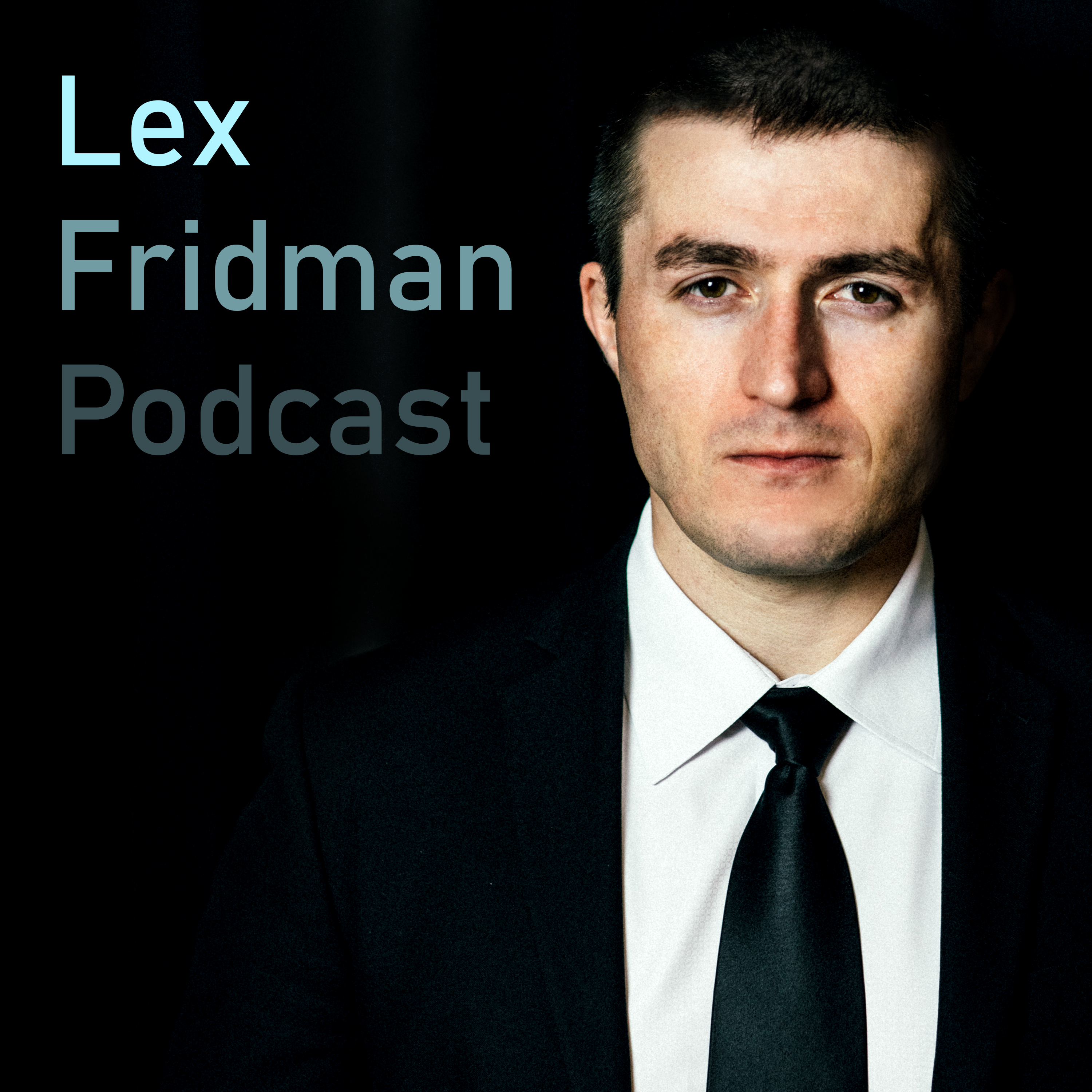
The Evolution of Language Models in Neural Networks
The conversation around language models in neural networks has shifted with the growth in data and compute, as empirically, larger language models show potential for understanding semantics without imposing a theory of language onto the learning mechanism. This change in trajectory has been evident in the recent work of OpenAI.
Clips
The field of deep learning is fortunate in its ability to produce unambiguous results, such as proving unproven theorems or solving open-ended problems.
55:11 - 56:45 (01:34)
Summary
The field of deep learning is fortunate in its ability to produce unambiguous results, such as proving unproven theorems or solving open-ended problems. Writing good code and solving complex problems without out-of-the-box solutions are also important skills in the field.
ChapterThe Evolution of Language Models in Neural Networks
Episode#94 – Ilya Sutskever: Deep Learning
PodcastLex Fridman Podcast
The history of neural networks in language and text changed with the advent of data and compute.
56:45 - 58:27 (01:41)
Summary
The history of neural networks in language and text changed with the advent of data and compute. The recent history of using neural networks in text has focused on language models.
ChapterThe Evolution of Language Models in Neural Networks
Episode#94 – Ilya Sutskever: Deep Learning
PodcastLex Fridman Podcast
The use of larger networks and greater compute power may lead to a future where language models are able to exhibit signs of semantic understanding, without requiring the imposition of a theory of language on the learning mechanism.
58:27 - 1:01:19 (02:52)
Summary
The use of larger networks and greater compute power may lead to a future where language models are able to exhibit signs of semantic understanding, without requiring the imposition of a theory of language on the learning mechanism.
ChapterThe Evolution of Language Models in Neural Networks
Episode#94 – Ilya Sutskever: Deep Learning
PodcastLex Fridman Podcast
The transformer algorithm is successful because it combines multiple ideas simultaneously, including attention, a great fit to the GPU, and being less deep, making it easier to optimize.
1:01:19 - 1:02:44 (01:25)
Summary
The transformer algorithm is successful because it combines multiple ideas simultaneously, including attention, a great fit to the GPU, and being less deep, making it easier to optimize. It allows achieving better results for the same amount of compute.
ChapterThe Evolution of Language Models in Neural Networks
Episode#94 – Ilya Sutskever: Deep Learning
PodcastLex Fridman Podcast
The advancement in the GANs technology made it possible to train big language models leading to a significant improvement in the samples.
1:02:45 - 1:04:16 (01:30)
Summary
The advancement in the GANs technology made it possible to train big language models leading to a significant improvement in the samples. The theory behind it predicted this improvement but witnessing it created a remarkable moment in AI development.