Chapter
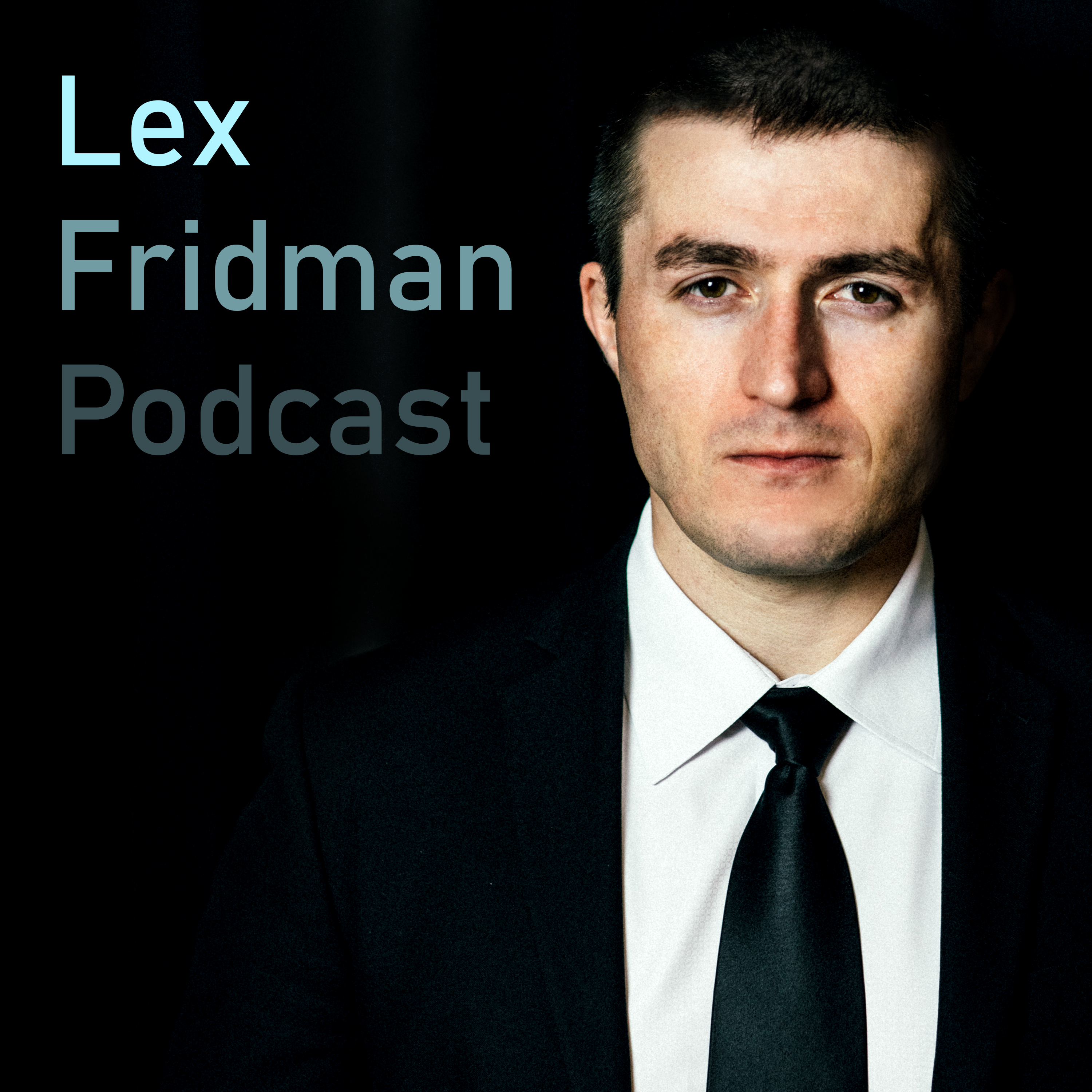
The Trade-Off Between Accuracy and Fairness in Predictive Models.
Predictive models that are trained to maximize overall accuracy will be less accurate among minority groups if the training data set is mainly composed of white males, pointing out the trade-offs between fairness and accuracy that often exist in these models.
Clips
The use of Pareto curves can help to balance concerns over racial fairness and accuracy in the criminal justice system.
36:24 - 42:35 (06:10)
Summary
The use of Pareto curves can help to balance concerns over racial fairness and accuracy in the criminal justice system. They can serve as a valuable tool for researchers and stakeholders to understand the trade-offs and pick a point to achieve reform.
ChapterThe Trade-Off Between Accuracy and Fairness in Predictive Models.
EpisodeMichael Kearns: Algorithmic Fairness, Bias, Privacy, and Ethics in Machine Learning
PodcastLex Fridman Podcast
The trade-off between accuracy and bias in machine learning often leads to models being trained on a majority group, which may lead to reduced accuracy on underrepresented groups.
42:35 - 45:13 (02:38)
Summary
The trade-off between accuracy and bias in machine learning often leads to models being trained on a majority group, which may lead to reduced accuracy on underrepresented groups. While gathering more data and training separate models for underrepresented groups can potentially avoid this bias, it may come with additional costs for the company developing the model.