Chapter
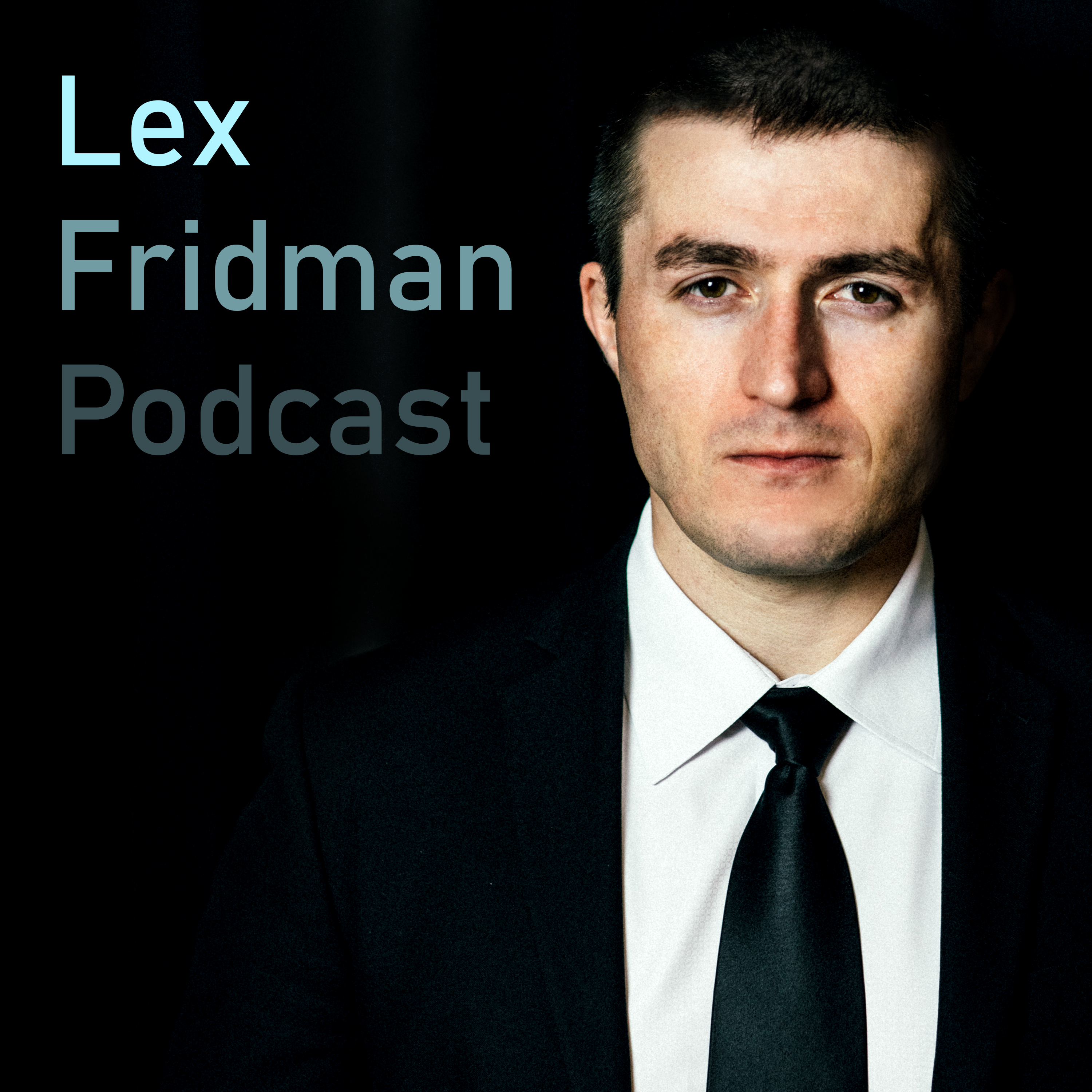
Understanding Causality in Neural Networks
Leon Battou and team have released a paper aimed at getting a neural net the ability to identify real causal relationships in order to address potential issues of data bias. This research will be important as it helps find a way to interpret knowledge and use it to minimize energy spent and optimize objective function.
Clips
The podcast discusses the role of energy minimization in optimizing an objective function for planning, and challenges the traditional approach of representing knowledge as logic systems.
17:14 - 21:40 (04:26)
Summary
The podcast discusses the role of energy minimization in optimizing an objective function for planning, and challenges the traditional approach of representing knowledge as logic systems.
ChapterUnderstanding Causality in Neural Networks
EpisodeYann LeCun: Deep Learning, Convolutional Neural Networks, and Self-Supervised Learning
PodcastLex Fridman Podcast
This podcast episode discusses the issue of causality and how it relates to both intelligent systems and physics, including the notion of time reversal at the microscopic level and the emergence of an arrow of time.
21:40 - 23:31 (01:50)
Summary
This podcast episode discusses the issue of causality and how it relates to both intelligent systems and physics, including the notion of time reversal at the microscopic level and the emergence of an arrow of time. Additionally, recent research on getting neural networks to truly understand real causal relationships is discussed.
ChapterUnderstanding Causality in Neural Networks
EpisodeYann LeCun: Deep Learning, Convolutional Neural Networks, and Self-Supervised Learning
PodcastLex Fridman Podcast
The podcast discusses the role of common sense and understanding of physics in deciphering causality, the reason for the loss of faith in deep learning during the 90s, and the discovery of deep learning over a decade later.
23:31 - 25:28 (01:57)
Summary
The podcast discusses the role of common sense and understanding of physics in deciphering causality, the reason for the loss of faith in deep learning during the 90s, and the discovery of deep learning over a decade later. It highlights the wrong causal relationships that have been attributed to deity or divinity over centuries.