Chapter
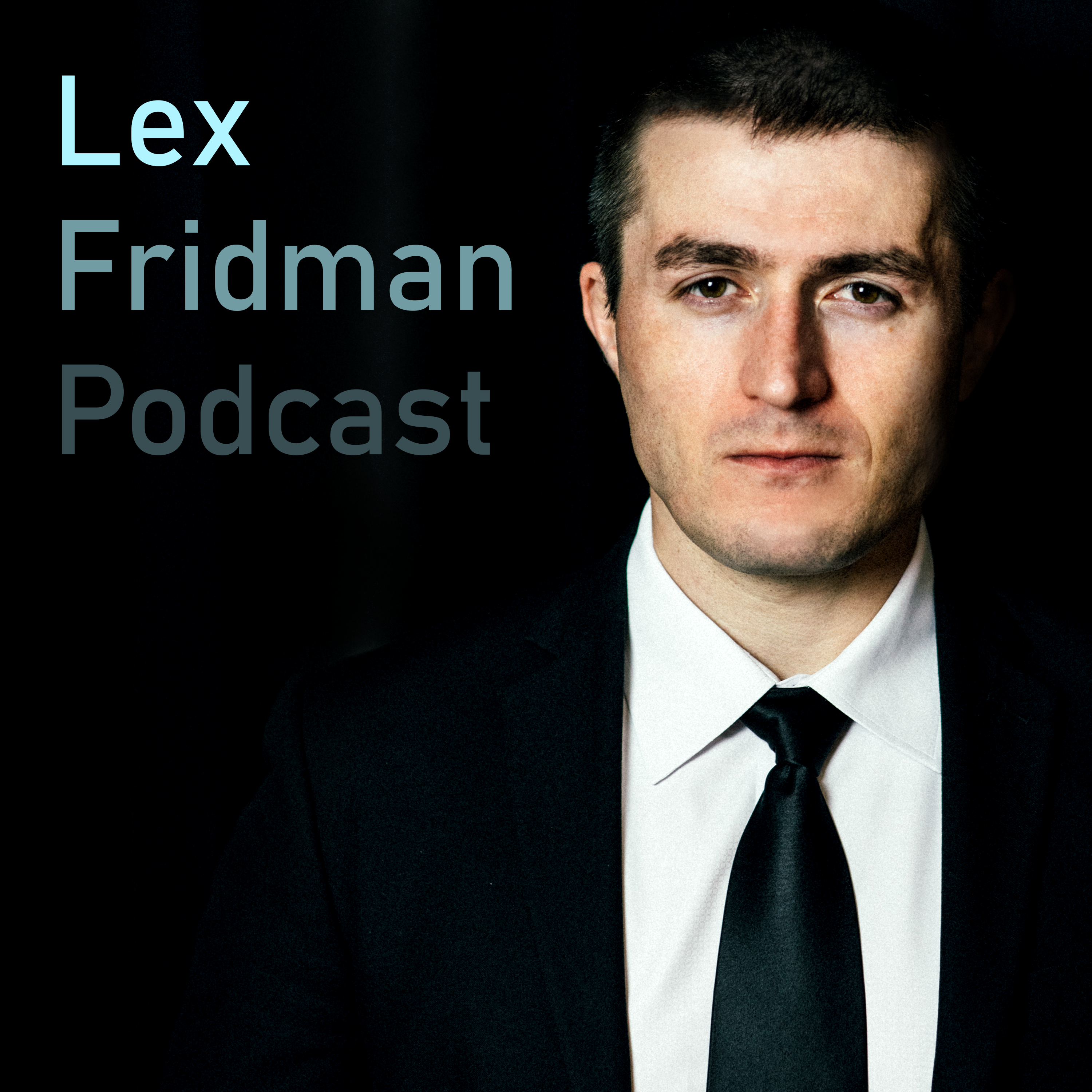
Understanding Cause and Effect Relationships
The absence of mathematical equations to capture the idea of cause and effect relationships makes it challenging to handle models without them. Deep neural networks can be conceptualized as conditional probability estimators that help infer cause and effect relationships.
Clips
The conversation explores the idea that while machine learning excels in making predictions based on data, it falls short in explaining causality.
22:21 - 23:42 (01:20)
Summary
The conversation explores the idea that while machine learning excels in making predictions based on data, it falls short in explaining causality. The need to find a more causal approach in machine learning is discussed.
ChapterUnderstanding Cause and Effect Relationships
EpisodeJudea Pearl: Causal Reasoning, Counterfactuals, Bayesian Networks, and the Path to AGI
PodcastLex Fridman Podcast
In order to infer cause and effect relationships in data, a human expert is needed to specify the initial model.
23:42 - 26:10 (02:27)
Summary
In order to infer cause and effect relationships in data, a human expert is needed to specify the initial model. By identifying which variables are "listening" to one another, we can better understand the relationships within the data.