Episode
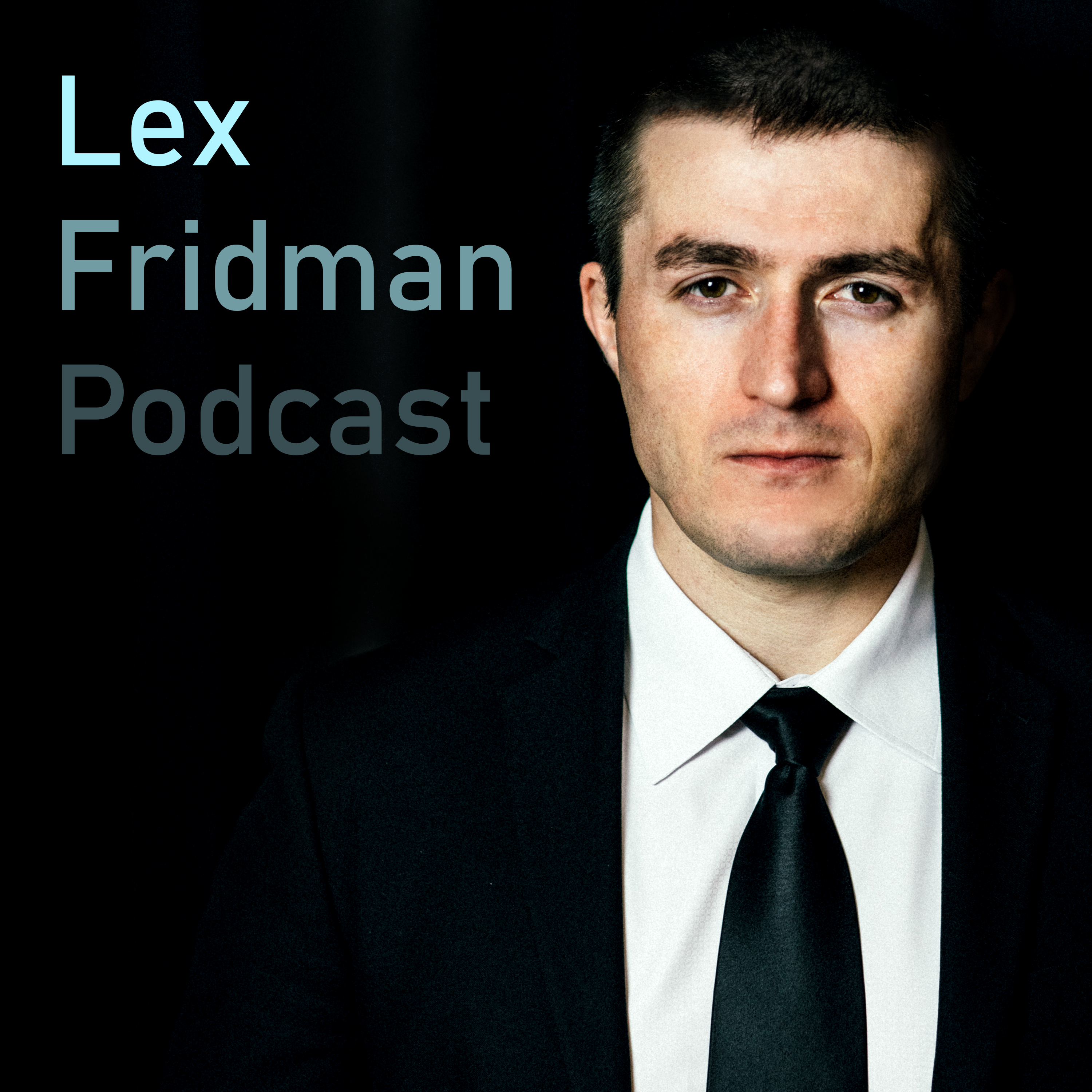
Yoshua Bengio: Deep Learning
Description
Yoshua Bengio, along with Geoffrey Hinton and Yann Lecun, is considered one of the three people most responsible for the advancement of deep learning during the 1990s, 2000s, and now. Cited 139,000 times, he has been integral to some of the biggest breakthroughs in AI over the past 3 decades. Video version is available on YouTube. If you would like to get more information about this podcast go to https://lexfridman.com/ai or connect with @lexfridman on Twitter, LinkedIn, Facebook, or YouTube where you can watch the video versions of these conversations.
Chapters
The mismatch between artificial neural nets and biological plausibility is an interesting area of study for understanding how brains work and for developing new ideas on how to incorporate the differences into artificial neural nets.
00:00 - 07:48 (07:48)
Summary
The mismatch between artificial neural nets and biological plausibility is an interesting area of study for understanding how brains work and for developing new ideas on how to incorporate the differences into artificial neural nets. Training neural nets to focus on causal explanations is an area where progress can be made.
EpisodeYoshua Bengio: Deep Learning
PodcastLex Fridman Podcast
The advancement of artificial intelligence research into active agents that learn by intervening in the world and the development of objective functions that allow for high-level explanations to emerge from the learning process present new and exciting opportunities for academics to advance the state of the art, particularly in training frameworks, learning models, and agent learning in synthetic environments, using projection of data into the right semantic space.
07:48 - 20:28 (12:39)
Summary
The advancement of artificial intelligence research into active agents that learn by intervening in the world and the development of objective functions that allow for high-level explanations to emerge from the learning process present new and exciting opportunities for academics to advance the state of the art, particularly in training frameworks, learning models, and agent learning in synthetic environments, using projection of data into the right semantic space.
EpisodeYoshua Bengio: Deep Learning
PodcastLex Fridman Podcast
The public discussion on AI and its impact should focus more on the short-term and medium-term negative effects like security, job market, concentration of power, discrimination and social issues which could threaten democracy, rather than always painting the picture of AI as a loose, killing machine or super intelligence that will destroy humanity.
20:28 - 25:45 (05:16)
Summary
The public discussion on AI and its impact should focus more on the short-term and medium-term negative effects like security, job market, concentration of power, discrimination and social issues which could threaten democracy, rather than always painting the picture of AI as a loose, killing machine or super intelligence that will destroy humanity.
EpisodeYoshua Bengio: Deep Learning
PodcastLex Fridman Podcast
The concept of machine teaching has been coined to emphasize the need for attention to be paid to the process of teaching machine learning systems.
25:45 - 35:17 (09:31)
Summary
The concept of machine teaching has been coined to emphasize the need for attention to be paid to the process of teaching machine learning systems. As more human-machine interaction emerges, it is necessary to build unbiased systems and instill a fundamental respect for human beings in the machines.
EpisodeYoshua Bengio: Deep Learning
PodcastLex Fridman Podcast
The challenges in building machine learning systems that can understand language and causal relationships in the world are still significant, but the future of these systems lies in model-based RL and building models that can better generalize to new distributions.
35:17 - 42:55 (07:38)
Summary
The challenges in building machine learning systems that can understand language and causal relationships in the world are still significant, but the future of these systems lies in model-based RL and building models that can better generalize to new distributions. Language differences are minute in the grand scheme and the goal is to create systems that can learn from human agents regardless of their language.