Chapter
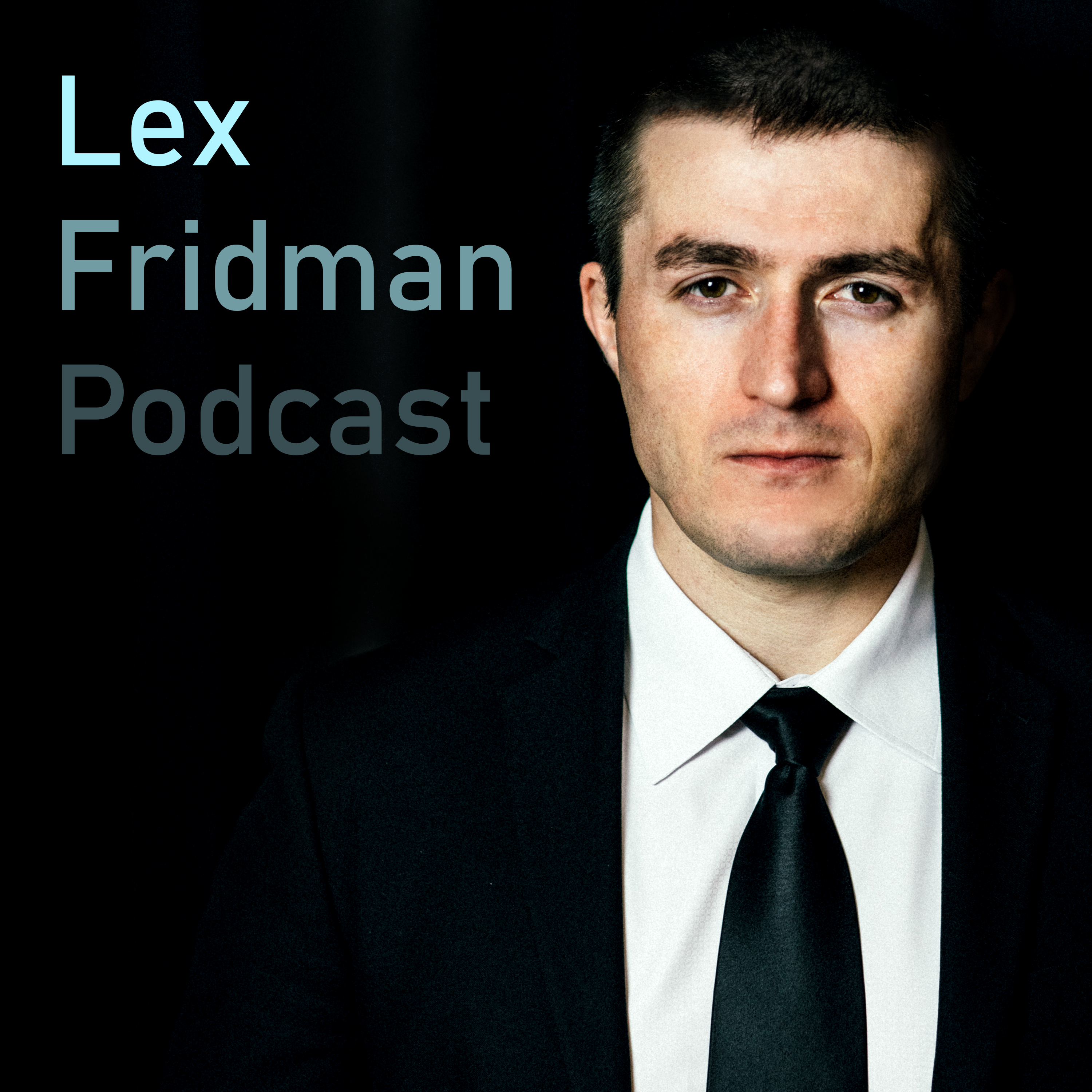
Building AI Systems to Make the World a Better Place
The speaker advocates for the use of AI to make the world a better place by highlighting applications in science like protein folding. They discuss the need to consider human values when building AGI systems that would eventually be able to do what they want.
Clips
The development of Artificial General Intelligence (AGI) can have great benefits for humanity but it is important to consider what values and goals should be integrated into AGI as we develop it.
1:34:52 - 1:36:45 (01:53)
Summary
The development of Artificial General Intelligence (AGI) can have great benefits for humanity but it is important to consider what values and goals should be integrated into AGI as we develop it. It is important to ask what it would look like for things to go right in the development of AGI.
ChapterBuilding AI Systems to Make the World a Better Place
Episode#106 – Matt Botvinick: Neuroscience, Psychology, and AI at DeepMind
PodcastLex Fridman Podcast
The speaker highlights the need for interdisciplinary studies in AI development, including reading literature from fields such as economics and social choice theory, in order to understand how humans can be brought into contact with AI systems in a way that improves the world.
1:36:45 - 1:40:01 (03:15)
Summary
The speaker highlights the need for interdisciplinary studies in AI development, including reading literature from fields such as economics and social choice theory, in order to understand how humans can be brought into contact with AI systems in a way that improves the world.
ChapterBuilding AI Systems to Make the World a Better Place
Episode#106 – Matt Botvinick: Neuroscience, Psychology, and AI at DeepMind
PodcastLex Fridman Podcast
Distributional reinforcement learning is a new version of reinforcement learning that uses a distribution of possible outcomes rather than a single expected value, allowing for faster and better policy learning.
1:40:01 - 1:43:31 (03:29)
Summary
Distributional reinforcement learning is a new version of reinforcement learning that uses a distribution of possible outcomes rather than a single expected value, allowing for faster and better policy learning.